Keynote 1
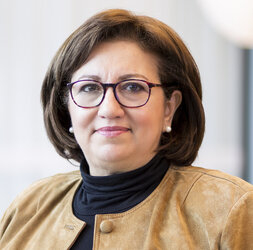
Nozha Boujemaa
Digital Trust Officer, Decathlon
Keynote title: How Responsible LLMs are beneficial to search and exploration in Retail industry
Abstract
In this talk, we first introduce responsible and trustworthy AI principles according to international standards and present OECD.AI effort to build interoperable AI risk management frameworks developed by existing standards. We present our approach to implement Trustworthy AI in a measurable way to build the proof of Digital Trust also how to make it happen through organizational way in an industrial setting.
In the second part of this talk, we present our approach to build responsible Generative AI models in retail which allow better understanding of customers’ needs while building richer indexing and search mechanism that extract hidden connections. We have more control on our LLM model being trained on business product range but also embedding user control and human agency interaction by design. It provides better interpretability and privacy-enhancing approach which minimize the user profiling. We will be presenting a demo of an R&D prototype in this regard able to provide insights and sales steerability with a multimodal approach. This prototype shows the proof of concept of responsible LLM development.
This is why we argue that we should strengthen the development of safe and responsible GenAI and GPT models rather than pausing their development.
Short Bio
Nozha Boujemaa is appointed as Digital Trust Officer at Decathlon. She was the Global Vice President Digital Ethics & Responsible AI for IKEA-Retail where she was focused on operationalizing trustworthy AI across IKEA’s retail operations in over 30 countries.
Dr. Boujemaa was Vice-Chair of the European Commission’s High Level Expert Group on Artificial Intelligence, where she led the development of the “Ethical Guidelines for Trustworthy AI.” She also coordinated the work on Trustworthy AI principles at the OECD and was co-chair of the “OECD.AI Network of Experts working group on implementing trustworthy AI.” Today, she is co-chair of the “OECD.AI Expert Group on Risk & Accountability”, where she translates these principles into actions. In addition, she is a member of the World Economic Forum’s Steering Committee on Digital Trust.
Dr. Boujemaa founded the interdisciplinary AI institute DATAIA and was the director of Inria Saclay Research Center, where she also supervised more than 25 PhDs in computer vision and search engines. She has also held numerous positions leading teams in areas such as large-scale information retrieval in media archives, security, earth observation and biodiversity. Prior to joining Ingka to operationalize trustworthy AI principles, Dr. Boujemaa served as Chief Science and Innovation Officer at a personalised medicine company using AI for early diagnosis and cancer prediction in the healthcare industry.
Dr. Boujemaa holds a PhD and HDR in Computer Science, is a Knight of the French National Order of Merit, and a proud mother of a data scientist and a data analyst, as well as the wife of a digital strategist.
Keynote 2
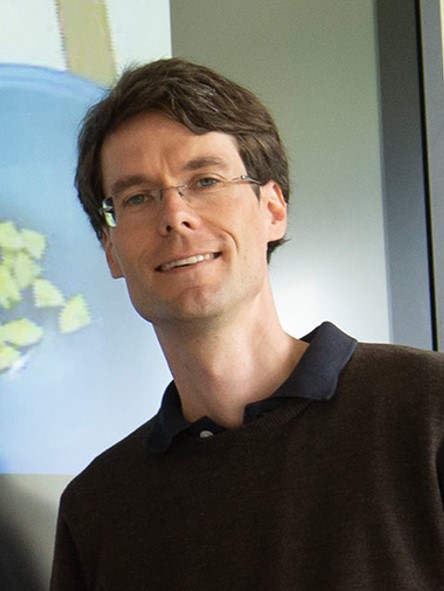
Prof. Dr. Jürgen Gall
Computer Vision Group, Department of Information Systems and Artificial Intelligence, University of Bonn
Keynote title: Efficient CNNs and Transformers for Video Understanding and Image Synthesis
Abstract
In this talk, I will first discuss approaches that reduce the GFLOPs during inference for 3D convolutional neural networks (CNN) and vision transformers. While state-of-the-art 3D CNNs and vision transformers achieve very good results on action recognition datasets, they are computationally very expensive and require many GFLOPs. While the GFLOPs of a 3D CNN or vision transformer can be decreased by reducing the temporal feature resolution or the number of tokens, there is no setting that is optimal for all input clips. I will therefore discuss two differentiable sampling approaches that can be plugged into any existing 3D CNN or vision transformer architecture. The sampling approaches adapt the computational resources to the input video such that as much resources as needed but not more than necessary are used to classify a video. The approaches substantially reduce the computational cost (GFLOPs) of state-of-the-art networks while preserving the accuracy. In the second part, I will discuss an approach that generates annotated training samples of very rare classes. It is based on a generative adversarial network (GAN) that jointly synthesizes images and the corresponding segmentation mask for each image. The generated data can then be used for one-shot video object segmentation.
Short Bio
Prof. Dr. Juergen Gall is professor and head of the Computer Vision Group at the University of Bonn since 2013, spokesperson of the Transdisciplinary Research Area “Mathematics, Modelling and Simulation of Complex Systems”, and member of the Lamarr Institute for Machine Learning and Artificial Intelligence. After his Ph.D. in computer science from the Saarland University and the Max Planck Institute for Informatics, he was a postdoctoral researcher at the Computer Vision Laboratory, ETH Zurich, from 2009 until 2012 and senior research scientist at the Max Planck Institute for Intelligent Systems in Tübingen from 2012 until 2013. He received a grant for an independent Emmy Noether research group from the German Research Foundation (DFG) in 2013, the German Pattern Recognition Award of the German Association for Pattern Recognition (DAGM) in 2014, an ERC Starting Grant in 2016, and an ERC Consolidator Grant in 2022. He is further spokesperson of the DFG funded research unit “Anticipating Human Behavior” and PI of the Cluster of Excellence “PhenoRob – Robotics and Phenotyping for Sustainable Crop Production”.
Slides
Keynote 3
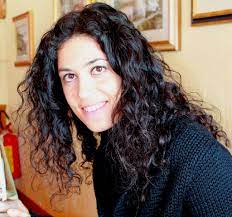
Elisa Ricci
Associate Professor at Department of Information Engineering and Computer Science (DISI) at the University of Trento
Keynote title: Recognizing actions in videos under domain shift
Abstract
Action recognition, which consists in automatically recognizing the action being performed in a video sequence, is a fundamental task in computer vision and multimedia. Supervised action recognition has been widely studied because of the growing need for automatically categorizing video content that are being generated everyday. However, it is nearly impossible for human annotators to keep pace with the enormous volumes of online videos, and thus supervised training becomes infeasible. A cheaper way of leveraging the massive pool of unlabelled data is by exploiting an already trained model to infer the labels on such data and then re-using them to build an improved model. Such an approach is also prone to failure because the unlabelled data may belong to a data distribution that is different from the annotated one. This is often referred to as the domain-shift problem. To address the domain-shift, recently Unsupervised Video Domain Adaptation (UVDA) methods have been proposed. However, these methods typically make strong and unrealistic assumptions. In this talk I will present some recent works of my research group on UVDA, showing that, thanks to recent advances in deep architectures and to the advent of foundation models, it is possible to deal with more challenging and realisting settings and recognize out-of-distribution classes.
Short Bio
Prof. Elisa Ricci (PhD, University of Perugia 2008) is an Associate Professor at Department of Information Engineering and Computer Science (DISI) at the University of Trento and the head of the Deep Visual Learning research group at Fondazione Bruno Kessler. She has published over 160 papers on international venues. Her research interests are mainly in the areas of computer vision, robotic perception and multimedia analysis. At UNITN she is the Coordinator of the Doctoral Programme in Information Engineering and Computer Science. She is an Associate Editor of IEEE Trans. on Multimedia, Computer Vision and Image Understanding and Pattern Recognition. She was the Program Chair of ACM MM 2020 and the Diversity Chair of ACM MM 2022. She is the recipient of the ACM MM 2015 Best Paper award and ICCV 2021 Honorable mention award.